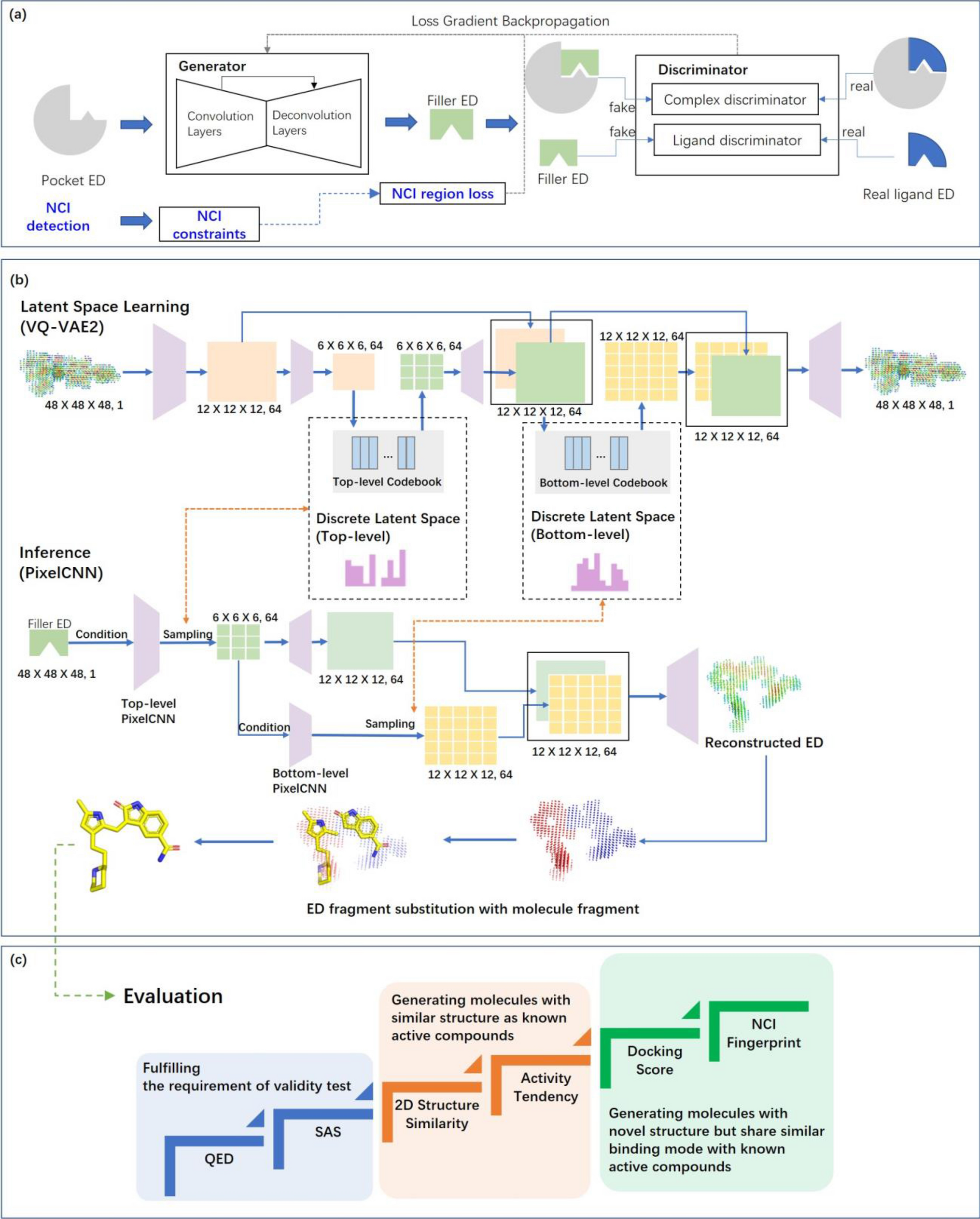
2022年9月6日,望石团队的原创研究成果“A pocket‑based 3D molecule generative model fueled by experimental electron density”在Scientific Reports上发表。此项工作是望石团队继首次提出大分子实验电子密度在AI领域应用的理论基础后的一项技术成果。该分子生成模型充分的利用实验电子密度中所包含的口袋与配体之间的互补信息,帮助小分子创新药设计人员更加高效的应对“基于靶点口袋结构设计小分子”的课题。具体来说,该模型所生成的分子结构和电子密度可以为药物设计人员提供“理想配体的形状、骨架、与受体的关键相关作用”等重要信息。
参见:
望石智慧报道 - https://mp.weixin.qq.com/s/vAnzWEcoFFyGnKulEwH6Dw
论文原文 - https://doi.org/10.1038/s41598-022-19363-6
论文摘要:
We report for the first time the use of experimental electron density (ED) as training data for the generation of drug-like three-dimensional molecules based on the structure of a target protein pocket. Similar to a structural biologist building molecules based on their ED, our model functions with two main components: a generative adversarial network (GAN) to generate the ligand ED in the input pocket and an ED interpretation module for molecule generation. The model was tested on three targets: a kinase (hematopoietic progenitor kinase 1), protease (SARS‐CoV‐2 main protease), and nuclear receptor (vitamin D receptor), and evaluated with a reference dataset composed of over 8000 compounds that have their activities reported in the literature. The evaluation considered the chemical validity, chemical space distribution-based diversity, and similarity with reference active compounds concerning the molecular structure and pocket-binding mode. Our model can generate molecules with similar structures to classical active compounds and novel compounds sharing similar binding modes with active compounds, making it a promising tool for library generation supporting high-throughput virtual screening. The ligand ED generated can also be used to support fragment-based drug design. Our model is available as an online service to academic users via https://edmg.stonewise.cn/#/create.